Artificial Intelligence is revolutionizing food processing businesses from Farm to Fork by intelligently identifying bottlenecks, event triggers, informing decision making, selectively allocating resources through Machine Learning pattern recognition.
This is done by meaningful data collection, refining elements such as timing process steps, targeting specific people skills, human capital support, functional technology, risk identification and rationalised controls. Artificial Intelligence (AI) is radically boosting efficiency gains and profitability for companies who make this R&D leap, an early and long term learning strategy.
If you are a high-performance manager, hold your breath and let’s jump straight in.
Recently the Food and Drug Administration (FDA) joined a number of organisations and food processing companies globally, rolling out the first of several Artificial Intelligence (AI) pilot programmes that use Machine Learning (ML) in its food import-screening processes.
The FDA state that Machine Learning now makes it possible to “rapidly analyze data, identifying connections and patterns that people or even its current rules-based screening system cannot see”.
So! What can Artificial Intelligence Algorithms tell us that we don’t already know or that ‘people cannot see’?
People are not perfectly designed for complex analysis. Our human brains have a limiting factor – our capacity for short-term memory is limited to about seven to fifteen ‘chunks’ of short-term information we can hold on to at any one time.
People are not perfectly designed for complex analysis. Our human brains have a limiting factor – our capacity for short term memory is limited to about seven chunks of short-term memory at a time.
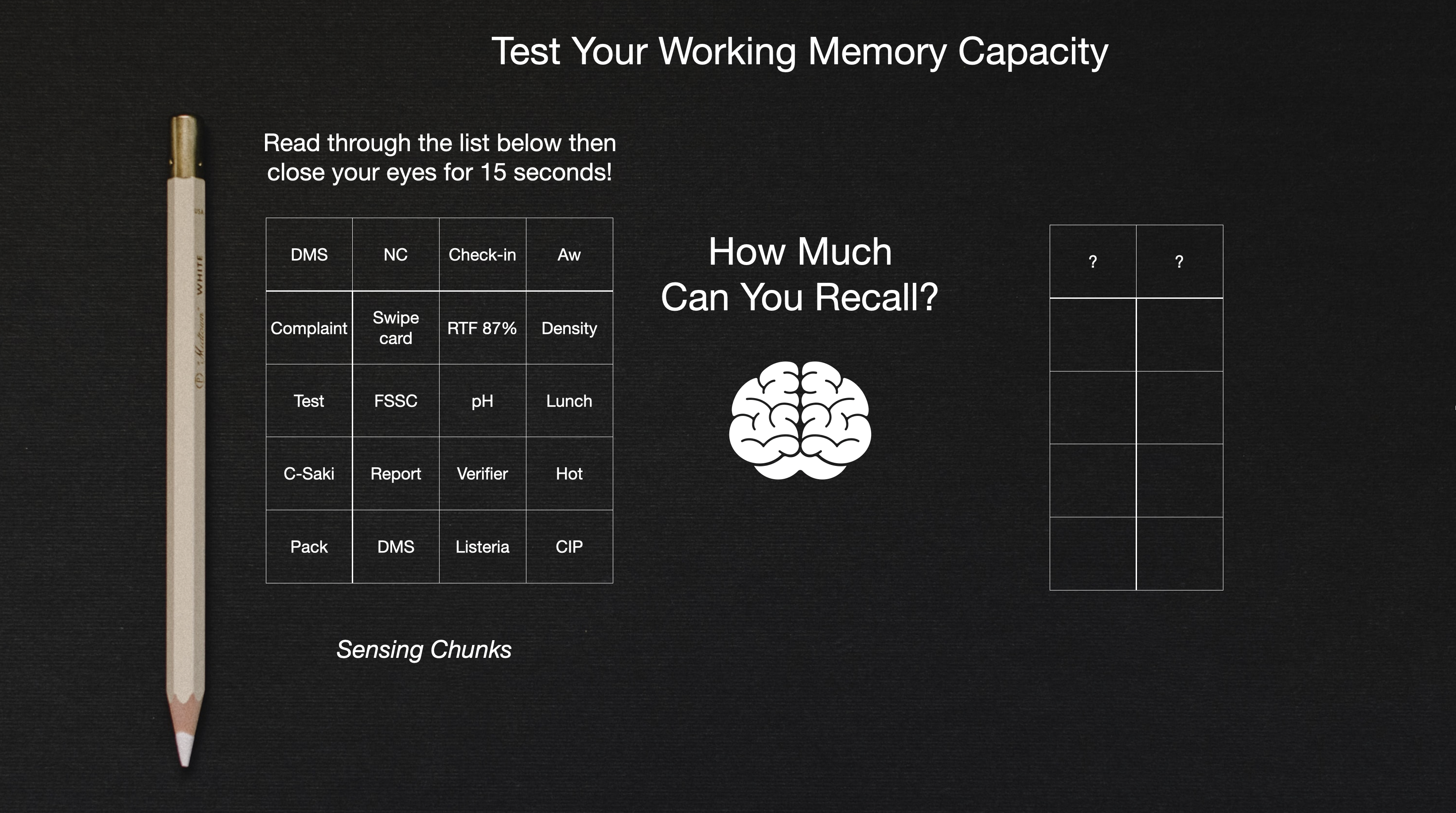
What does this limited short-term memory mean to a person or a food business?
This means that if you are a Chief Executive or a General Manager (GM) – Food Safety & Quality Assurance – and you have about 25 chunks of information and data points, being thrown at you from a summary of the Daily Management System (DMS) meetings today, this information is stored temporarily in the prefrontal cortex of your brain for about 15 seconds. The number of information chunks the average manager could cognitively hold on to and remember in our working memory is between 7 to 15.
This limits our ability as people and company management, to first hold (explained in the example above), then remember and finally based on our further declining long term memory retention capacity, intelligently analyse what is still only part of the full spectrum of food processing information.
This reduces our ability to recognise the complex patterns within the food processing enviroment and where Artificial Intelligence and Machine Learning comes in. Algorithms are not limited in such a way.
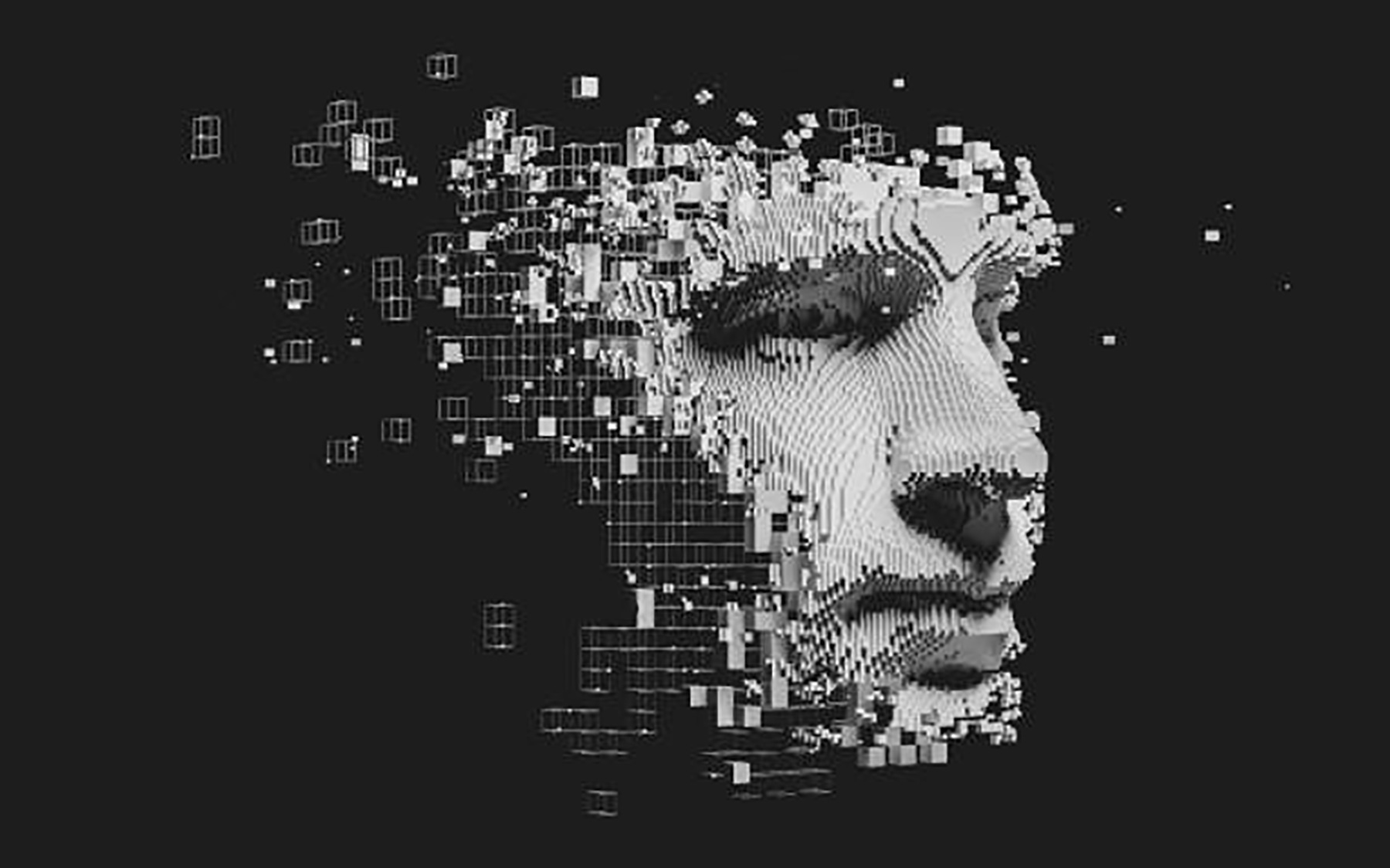
Artificial Intelligence pattern recognition and understanding the difference between the terms Causation and Correlation
Simply put, causation is linked to a cause-and-effect situation where an independent variable (condition or piece of information or something we do in a food business) has a direct impact on the dependent variable (condition or piece of information in a food business that is controlled or influenced by an independent variable) or a final processed food product.
A correlation, however, is a relationship between two variables. An independent variable (condition or piece of information in a food business) that can be controlled or changed that affects a dependent variable (condition or piece of information in a food business that is controlled or influenced by an outside factor, most often the independent variable). Generally speaking, correlations are not immediately obvious to people or food business because of our inherent human limitations explained above.
Understanding how Artificial Intelligence is Revolutionizing Processing on the Farm
The story I’m about to describe is about a dairy farm in Japan that deployed artificial intelligence toward process efficiency.
It’s a GYUHO SaaS Fujitsu AI solution also referenced by Thomas Friedman in his book, Thank you for being late.
This blog attempts to better describe and detail how Artificial Intelligence works and how machine learning in this type of project is practically applied in the food chain.
Static and Dynamic Data (Information) Collection in Food Processing
To begin with, let’s understand how data is collected in food companies. Data on a farm similar to food manufacturing companies is collected in Static and Dynamic forms.
In this case, the farm was fairly advanced, in terms of static data collection features. Examples of static data include: feed quantity, number of cows or production capacity (independent variables) product (milk) volumes and product temperature etc (dependent variables) and the resulting pattern recognition was put to good use for the rationalised efficiency-focused decision making with further process optimisation.
The revolutionary intelligent insight for this farm example that follows came from a dynamic data collection system in the form of pedometers Fijutsu decided to fit on a herd of cows.
Now if I said to you I was going to put a pedometer wristband on your hand, what you could think is (if you were Daisy the cow then) what the analyst is trying to understand from the information gained from the pedometer is to track the steps taken and potentially relate this to the volume of milk produced etc.
If you thought the pedometer would be used the same way as one that tracks exercise and calories burnt such as on a smartwatch, though entirely plausible, is not where the true unbound power of artificial intelligence and machine learning pattern recognition can make an impact.
It’s easy to fall into the trap of thinking artificial intelligence and machine learning is about obvious cause and effect, however, its true power comes from also simultaneously correlating what independent variables and features are changing (outside of our limited human capacity to pattern-recognise) that’s potentially having an impact on a challenge we might be facing in a food business or in this case, the farm.
The challenge on the farm: Estrus
If you are a farmer, your herd of cows, let’s say Daisies, come into Estrus or heat during an 8 to 30-hour window, and sometimes this can inconveniently occur at night.
This means that if Daisy comes into Estrus in the middle of the night you have to grab your torch and find Daisy to fertilize her whatever the weather, rain, or snow and wherever she might be on the farm.
Importantly if we miss, or mistime, the opportunity to cross-fertilize Daisy then she won’t have a calf. If Daisy doesn’t have a calf, then Daisy won’t produce milk for us in the coming season. This is a problem the AI analyst identified as a gap that Artificial Intelligence could potentially provide dynamic pattern recognition insight into.
By way of background, cows produce milk for about 10 months after calving and then they are dried off for about sixty days before calving again in winter. So fertilisation has a year-long impact.
If you have a herd of, say, 100 Daisies, depending on how successful your artificial insemination process is, you only have a 60-80% average success ratio with cross-fertilization.
This means that you could possibly have 20 Daisies that might not become fertilized, might not have a calf, and might not produce milk on the farm in the coming season.
Think about that in terms of both milk production and the negative impact on annual productivity volumes (84,000 Lts) based on an average Daisy producing 4,200 litres of milk a year.
The average size of a dairy herd in New Zealand is about 500 cows.
The table below gives you an idea of both the scale and financial impact of inefficiencies or loss of production capacity.
Cows | KPI Metric (Production Volume) | Av Payout/lt | Financial Impact |
1 | 4,200 | $0.57 | $2,394 |
80 | 336,000 | $0.57 | $191,520 |
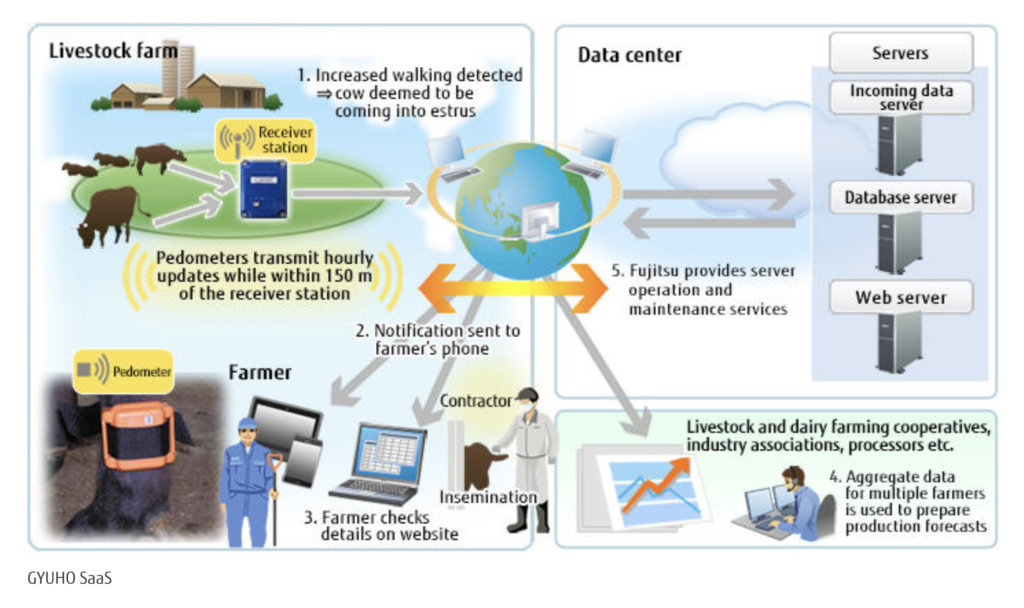
Artificial Intelligence pattern recognition and Dynamic Data data
Dynamic Date from pedometers: This is where artificial intelligence and machine learning truly cut the mustard for this specific case study outlined below.
The true success power of AI, in specifically the food sector comes, from when an analyst can work backwards from a problem rather than falling into the trap of starting with a pre-defined AI solution looking to find a problem it can fit.
The true success power of AI comes, from when an analyst can work backwards from a problem rather than falling into the trap of starting with a pre-defined AI solution looking to find a problem it can fit.
Understanding computer programmes versus artificial intelligence algorithms
It’s also important to understand that, generally speaking, computer systems are programmed with relatively restrictive and specific logical expressions. For example, if this is a condition then that is the pre-programmed instruction
What makes an algorithm machine learning different from a restrictive and predefined computer programme is it keeps learning by exposure to more and more food process information. A bit like you and I learnt from experience as kids!
What makes an algorithm machine learning different to a restrictive and predefined computer programme is it keeps learning by exposure to more and more food process information. A bit like you and I learnt from experience as kids!
AI algorithms create their own rules when it comes to pattern recognition and then further improve their intelligent insight by learning from additional experiences resulting from the application of these rules.
To throw more light on this ability of AI algorithms to create their own rules and build further pattern recognition intelligently this specific algorithm found that if the farmer cross-inseminates Daisy in the first four-hour window the farmer has a 70% chance of her calf being female and if the farmer inseminates Daisy in the second four-hour window there’s a higher chance of her calf being male.
So if you are a farmer, artificial intelligence helps you to now also shape your herd of either female calves for milk production or bobby calves for beef production.
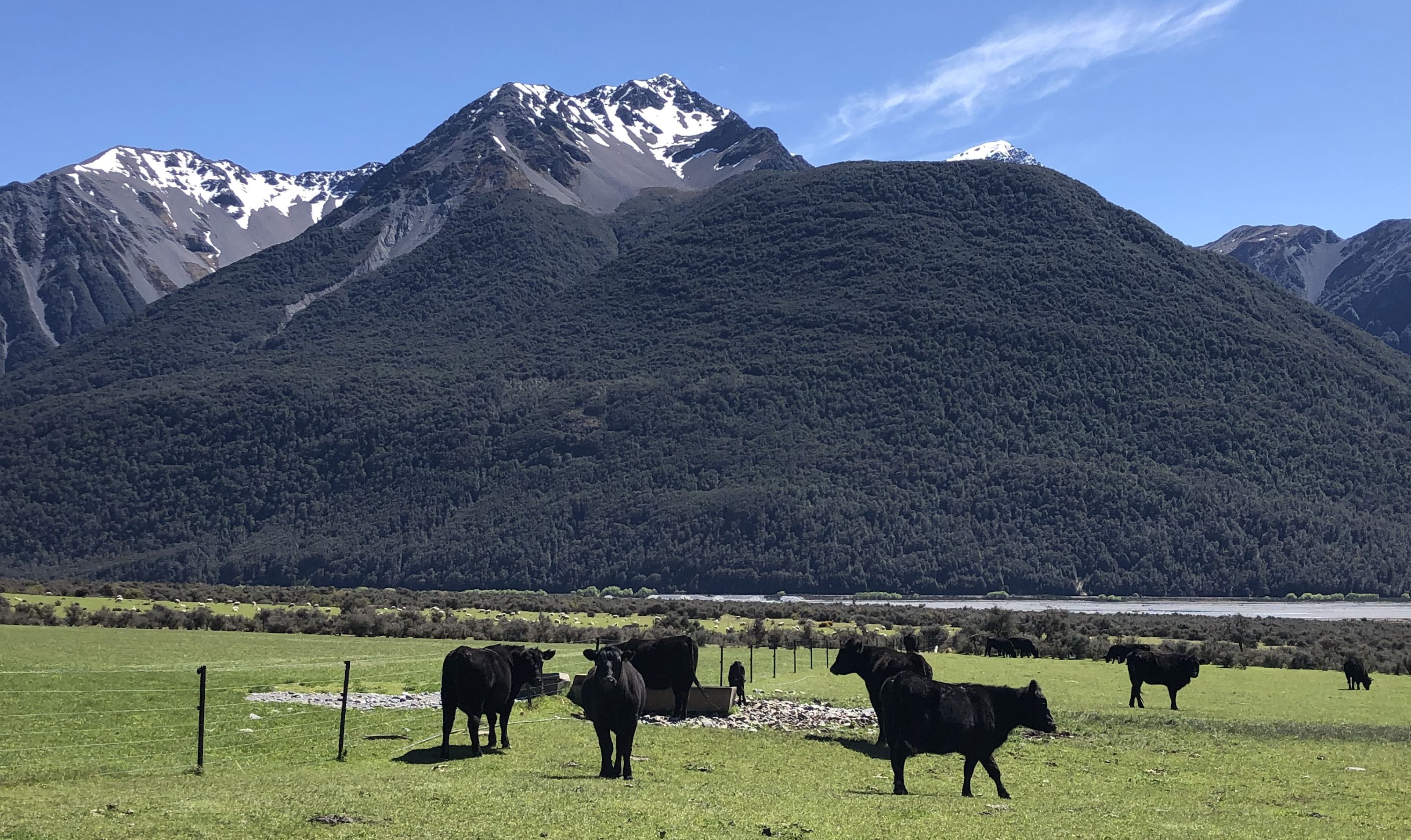
Now that we get how Artificial Intelligence is revolutionizing food processing businesses with an example on Farm above, here are even more ways Artificial Intelligence is revolutionizing the food chain, closer to Fork listed below:
What to watch out for | Current limiting factors | Revolutionary Artificial Intelligence Insight |
Next-generation sequencing (NGS) replaces the conventional characterisation of food-related pathogens eg Cronobacter Sakazakii with a genomic definition. | Routine microbiological surveillance might not show that a random illness/death or detection occurring over several months is related to a specific person, product or company. | NGS allows precise characterisation and potential traceability to a single operator/product/company. including previously unknown trigger features. |
Potential Big Data mining by Regulators to identify the patterns in food manufacture that correlate to the current high number of food recalls and process failures. | Current routine regulatory analysis may struggle to explain anomalies. | Big Data mining could uncover previously unknown trigger features in food manufacturing that correlate to food recalls and process failures that regulatory inspectors could then use to inform compliance verification. |
Prediction Dashboards identify the patterns in the food supply chain that correlate to Food Safety Management System (FSMS) Risks eg. Food Defence / Food Safety / Food Venerability. | Current routine QA analysis may not have the ability/resource to raise an FSMS risk alert in real-time. | AI-powered global Prediction Dashboards can identify the patterns and anomaly triggers in the global food supply chain that correlate to a Food Defence / Food Safety / Food Venerability risk and help inform management and a company’s auditing and testing protocols that can be resourced in real-time. |
Summary of learning
As discussed above, Artificial Intelligence is revolutionizing Food Processing Businesses from Farm to Fork by intelligent pattern recognition through Machine Learning, refining elements such as event triggers and timing process steps described with the example from a farm, and by doing so radically boosting efficiency gains and profitability of businesses willing to invest in the right Artificial Intelligence solutions across the food chain.
It’s important to recognise that no matter how knowledgeable and experienced we are, human beings are not perfectly designed for complex analysis, limiting our ability to first hold, then remember, and finally intelligently analyze the full spectrum of information that results in meaningful pattern recognition in a food-related process.
While all new technologies are challenging, this is still where Artificial intelligence and machine learning such as Food Safety Intelligence’s algorithms come into their own. What makes Food Safety Intelligence solutions both useful, as well as different, is algorithms keep learning by understanding more and more process information.
Continuous improvement, constant learning from across the many food sectors we work with coupled with our deep food safety experience and understanding are used to find meaningful features (process variable and things that change in your company).
Our Algorithms pattern recognizes what variables could relate to challenges your food company is facing, and by doing so provide you with the potential for gaining a highly competitive edge.
Food Safe’s artificial intelligence and machine learning capability from farm to fork includes intelligent solutions that could maximise the percentage of your food products processed Right-First-Time (RTF), as well as specific tracking of some other functions like metrics related to people, such as operator skills, hours worked, and functional technology (Quality Control data) – that are potentially impacting your product safety, staff attrition and food safety culture, simultaneously.
Author: Keith Michael.
Keith is Food Safe’s Managing Director and a Certified Company Director from the Institute of Directors (IOD), New Zealand. He assists companies across the Global Food Supply Chain with Artificial Intelligence, Food Safety Compliance and Risk Management with smart purpose-built solutions.
Keynote speaker: Food Safety Conferences in 2019, 2020 & 2021